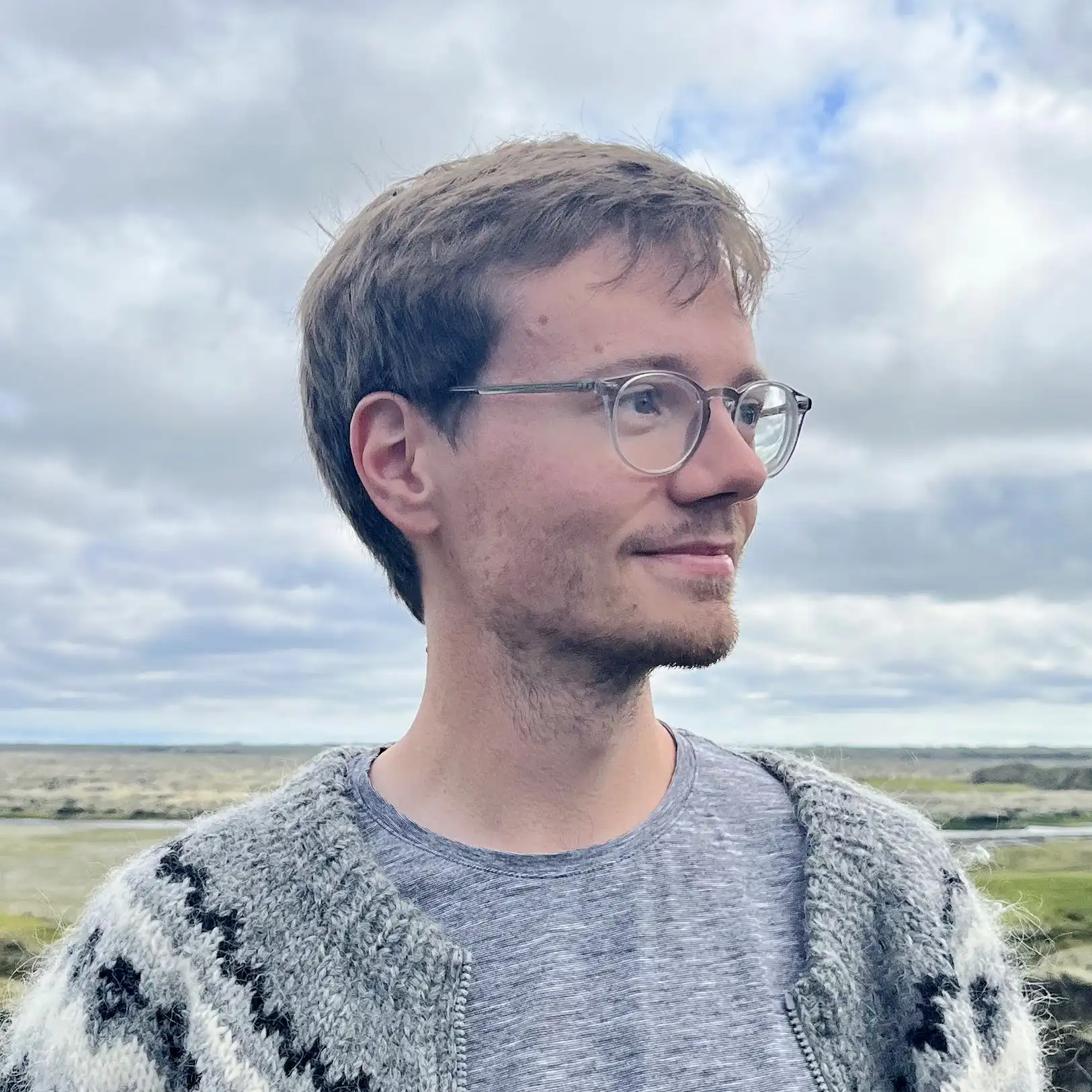
Arinbjörn Kolbeinsson
I advance machine learning research and transform deep technical innovations into practical solutions. My work spans developing efficient foundation models, applying deep learning to healthcare challenges and advancing AI applications in legal systems — bridging cutting-edge research with real-world impact.
Machine Learning Models and Systems
- Model interventions and editing for controllable AI
- 3D neural networks for medical computer vision
- Tensor methods for robust and efficient deep learning
Healthcare
Healthcare & Biomedicine
- Personalised health prediction using multi-modal data
- Systems for brain MRI analysis and systemic health markers
- Deep learning for time series analysis in healthcare
Legal
AI & Law
- Dynamic standardisation in legal contracts
- AI governance and regulatory frameworks
- Intersection of law and AI development
At the University of Virginia, I conduct research on Composable Interventions for Language Models, which has been accepted to ICLR 2025. My work develops frameworks to study how different types of interventions - like knowledge editing, model compression, and machine unlearning - interact when applied sequentially to the same model. With my collaborators, we have uncovered critical insights about intervention interactions and identified gaps in current approaches. This research aims to enhance model control and efficiency while maintaining performance, with implications for developing more robust multi-objective interventions.
At Evidation Health, I specialised in machine learning for health time series data. As part of the research team, I developed prediction models for FluSmart, a digital flu monitoring program that uses wearable sensor data to detect early signs of influenza-like illness. My research advanced the field through novel applications of self-supervised learning for time series analysis and development of generative models for wearable data. In collaboration with researchers from the University of Washington and Evidation, I contributed to Homekit2020, a large-scale public benchmark for wearable sensor data classification, containing over 14 million hours of multimodal health data. This work established new frameworks for analysing temporal health data and addressing data scarcity challenges in medical research.
During my PhD at Imperial College London, I developed novel deep learning approaches for biomarker discovery from
medical imaging and genomic data. My doctoral research introduced
3D-ResNet architectures for brain MRI analysis, achieving state-of-the-art accuracy in brain age prediction using over
21,000 scans from UK Biobank. Through innovative interpretation methods and phenome-wide association analysis, I
identified crucial links, and their causality, between brain structure and systemic health, particularly cardiometabolic and cognitive
conditions.
I also developed receptive field networks for analysing genome sequences, demonstrating how deep learning
can leverage both local and global genomic structure for polygenic risk prediction. This work bridges the gap between
raw medical data and actionable health insights through interpretable deep learning models.
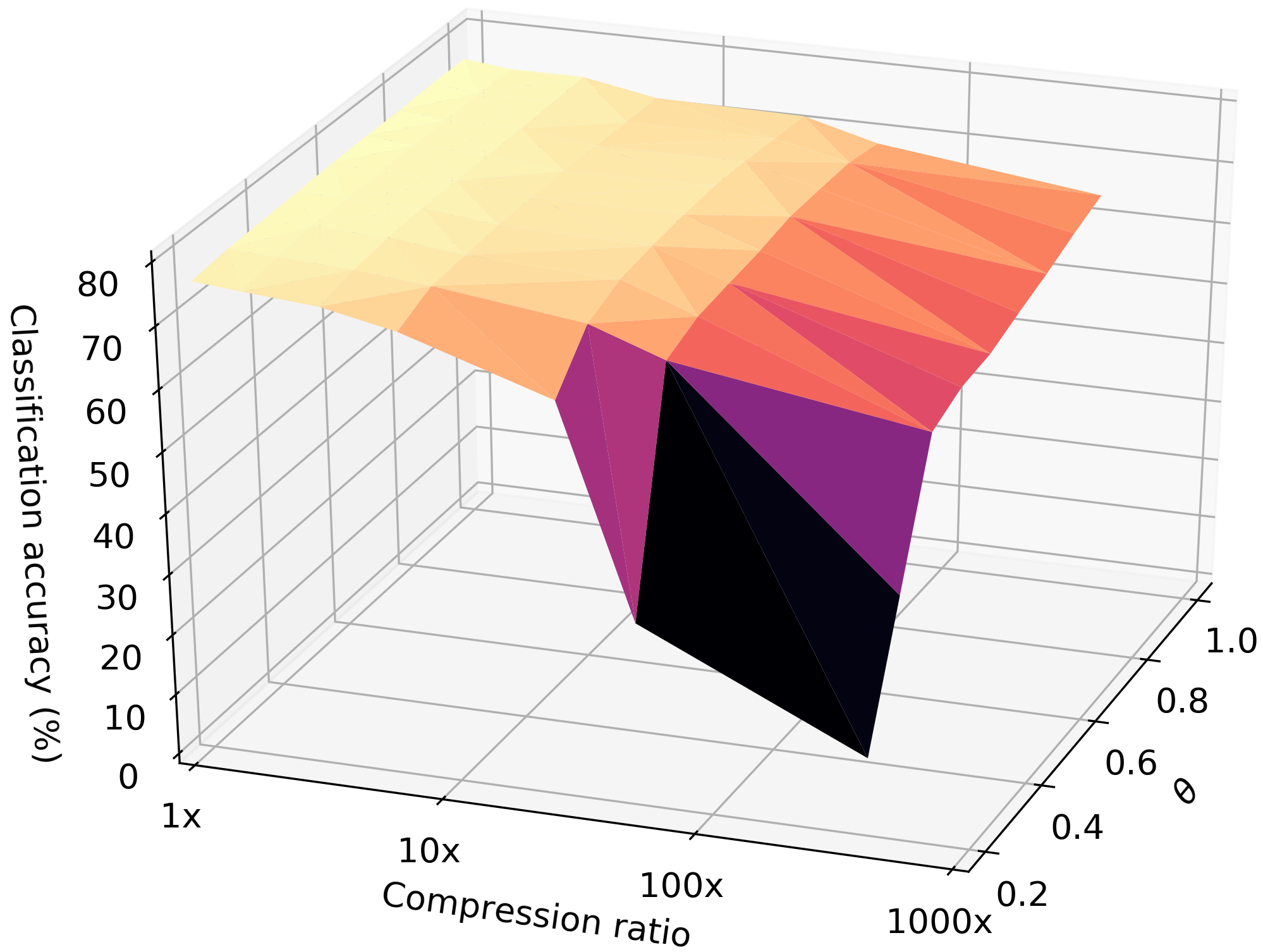
My research also focuses on tensor methods for deep learning, making neural networks more robust and efficient. I developed Tensor Dropout, a novel stochastic regularisation technique that significantly improves model robustness to both random and adversarial noise without requiring adversarial training. Through collaborations between Samsung AI and Caltech, I contributed to Tensor Regression Networks, demonstrating how tensor methods can reduce parameter count while preserving model expressiveness. These innovations were particularly impactful for medical imaging tasks, where they enabled more reliable predictions from 3D brain MRI data. My work established theoretical foundations for using tensor methods in deep learning while delivering practical performance improvements on real-world tasks.
Recently completed legal studies at the University of Iceland, where my thesis research focused on Dynamic Contract Standardisation (thesis in Icelandic) using AI systems. This work explored how machine learning can make standardised contracts more flexible while maintaining legal validity and protecting consumer rights. My combined expertise in AI development and law enables me to bridge technical innovation with regulatory frameworks, particularly in areas like contract automation and consumer protection. This interdisciplinary background provides unique insights into developing AI systems that are both technically sophisticated and legally compliant.
-
PhD: Deep learning for health outcome prediction
Imperial College London, 2021 -
MRes in Biomedical Research
Imperial College London, 2016 -
MEng in Bioengineering
Imperial College London, 2015 -
BA in Law
University of Iceland, 2024
- Arinbjörn Kolbeinsson, Kyle O'Brien, Tianjin Huang, Shanghua Gao, Shiwei Liu, Jonathan Richard Schwarz, Anurag Vaidya, Faisal Mahmood, Marinka Zitnik, Tianlong Chen and Thomas Hartvigsen. "Composable Interventions for Language Models". International Conference on Learning Representations (ICLR 2025).
- Mike A Merrill, Esteban Safranchik, Arinbjörn Kolbeinsson, Piyusha Gade, Ernesto Ramirez, Ludwig Schmidt, Luca Foschini, Tim Althoff. "Homekit2020: A benchmark for time series classification on a large mobile sensing dataset with laboratory tested ground truth of influenza infections". Conference on Health, Inference, and Learning (CHIL) (2023).
- Georgios Efstathiadis, Patrick Emedom-Nnamdi, Arinbjörn Kolbeinsson, Jukka-Pekka Onnela, Junwei Lu. "STASIS: Reinforcement Learning Simulators for Human-Centric Real-World Environments" Trustworthy Machine Learning for Healthcare (2023).
- Arinbjörn Kolbeinsson and Luca Foschini. "Generative models for wearables data." Workshop on Deep Generative Models for Health at NeurIPS (2023).
- Arinbjörn Kolbeinsson, Naman Shukla, Akhil Gupta, Lavanya Marla, and Kartik Yellepeddi. "Galactic Air Improves Ancillary Revenues with Dynamic Personalized Pricing." INFORMS Journal on Applied Analytics (2022).
- Arinbjörn Kolbeinsson, Jean Kossaifi, Yannis Panagakis, Adrian Bulat, Anima Anandkumar, Ioanna Tzoulaki, and Paul Matthews. "Tensor Dropout for Robust Learning," in IEEE Journal of Selected Topics in Signal Processing, doi: 10.1109/JSTSP.2021.3064182 (2021).
- Akhil Gupta, Lavanya Marla, Ruoyu Sun, Naman Shukla and Arinbjörn Kolbeinsson. “PenDer: Incorporating Shape Constraints via Penalized Derivatives”. In Proceedings of the AAAI Conference on Artificial Intelligence (2021).
- Arinbjörn Kolbeinsson, Sarah Filippi, Yannis Panagakis, Paul M. Matthews, Paul Elliott, Abbas Dehghan, and Ioanna Tzoulaki. "Accelerated MRI-predicted brain ageing and its associations with cardiometabolic and brain disorders." Scientific Reports 10, no. 1 (2020).
- Kossaifi, Jean, Zachary C. Lipton, Arinbjörn Kolbeinsson, Aran Khanna, Tommaso Furlanello, and Anima Anandkumar. "Tensor regression networks." Journal of Machine Learning Research 21, no. 123 (2020).
- See all on my Google Scholar profile.